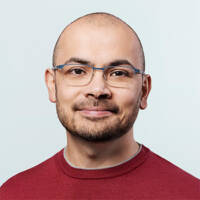
Demis Hassabis
Google DeepMind
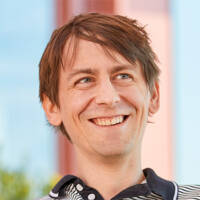
John Jumper
Google DeepMind
For the invention of AlphaFold, a revolutionary technology for predicting the three-dimensional structure of proteins
The 2023 Albert Lasker Basic Medical Research Award honors two scientists for the invention of AlphaFold, the artificial intelligence (AI) system that solved the long-standing challenge of predicting the three-dimensional structure of proteins from the one-dimensional sequence of their amino acids. With brilliant ideas, intensive efforts, and supreme engineering, Demis Hassabis and John Jumper (both of Google DeepMind, London) led the AlphaFold team and propelled structure prediction to an unprecedented level of accuracy and speed. This transformational method is rapidly advancing our understanding of fundamental biological processes and facilitating drug design.
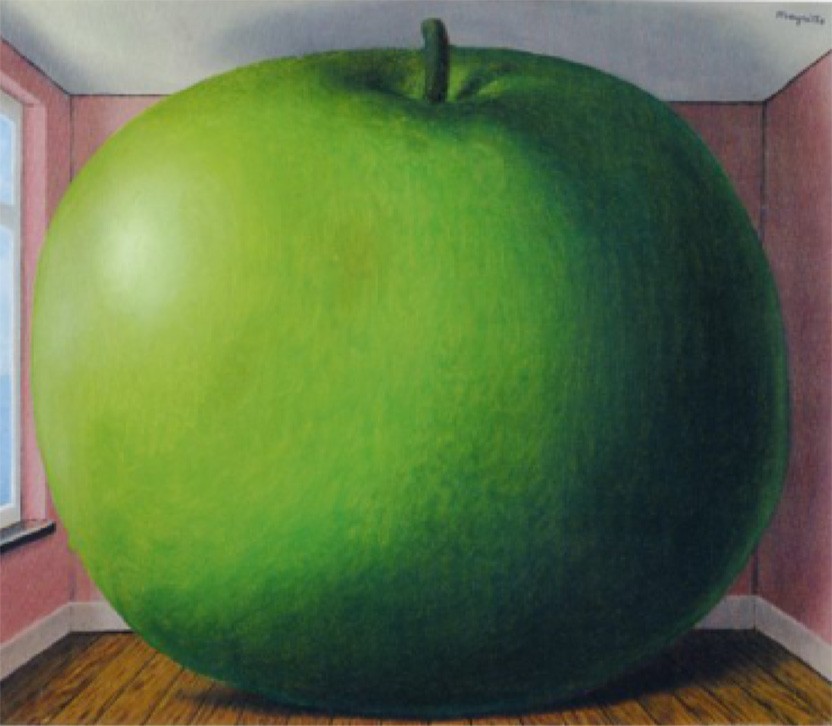
The Secret to a Successful Career in Science—According to Magritte
There is no shortage of words of advice on how to become a successful scientist.
Award Presentation: Erin O’Shea
Cells in our body rely on proteins to perform many different tasks, including digestion of our food, contraction of our muscles, and transmission of signals between neurons inside our brain. Proteins are made up of building blocks called amino acids that are joined to each other in a particular order like different colored beads on a string. The order of amino acids is unique to each protein and is specified by our DNA. For proteins to carry out their functions, they must fold into a three-dimensional shape in which some amino acids that are far from one another in linear sequence come close to each other when the protein adopts its final shape. The process by which a protein goes from beads on a linear string to a three-dimensional structure is called protein folding.
For many years scientists have worked to understand how proteins fold into their structures. What tells a protein how to fold properly? Are there machines inside cells that help proteins fold? Or is the information that specifies the folded structure of a given protein contained within the sequence of amino acids itself? Part of the answer to these questions came from a beautiful experiment that Christian Anfinsen performed in 1962 (ref. 1). He put a protein into a test tube and used chemicals to unfold it – to destroy its folded structure but to leave the sequence of amino acids intact. He then asked what would happen if he removed the chemicals that caused the protein to unfold – would the protein fold back into its characteristic structure or not? Anfinsen got a clear and surprising result from this experiment – when he removed the unfolding chemical, the protein returned to its characteristic shape. This told Anfinsen that, at least for the protein he was studying, the information that specifies what structure the protein will adopt is contained in the sequence of amino acids. For this work Anfinsen was awarded the Nobel Prize in 1972.
After Anfinsen’s experiment, the race was on to learn how to predict the folded structure of a protein from its sequence of amino acids. If this could be done, we might better understand the jobs that proteins perform inside of cells, learn how mutations cause disease, and more easily identify drugs that are able to interact with proteins and thereby treat diseases. Over the years scientists have labored to determine protein sequences and protein structures. This has been a time-consuming, costly, and labor-intensive process—one which has been able to reveal the structure of only a mere fraction of known proteins. From this work, scientists have learned that proteins with similar sequences of amino acids adopt similar folded structures, reinforcing the notion that there is a code relating protein sequence to structure.
In 1994 a community-based competition called CASP (which stands for Critical Assessment of Structure Prediction) was launched to identify the algorithms that could most accurately predict protein structure from protein sequence. In each annual competition, scientists are given sequences of test proteins whose structures are not known and are challenged to use computer-based methods to predict their structures. While the competition is happening, other scientists use experimental approaches to determine the structures of these test proteins. The winner is the scientist or group that can most accurately predict protein structures from protein sequences. For decades, scientists have made steady progress. But in the 2020 CASP competition, that all changed (ref. 2). The group at Google DeepMind led by John Jumper and Demis Hassabis generated structure predictions that were several-fold better than the other groups in the competition. In fact, many of the DeepMind predictions had accuracy comparable to those determined by experimental methods. After 30 years of competitions, the judges who assessed the model predictions declared the 50-year-old Anfinsen problem solved – most protein structures can be predicted from their sequences.
The team led by Jumper and Hassabis used their model to predict structures for more than 200 million protein sequences, which is already changing the practice of biology in labs worldwide.
So how did DeepMind do this? The short answer is that they built a computational model that relies on artificial intelligence or AI (ref. 3). But this is an oversimplification, as DeepMind was not the first to use AI approaches to address the protein structure prediction problem. So what made DeepMind’s approach so successful (ref. 4)? First, AI approaches make use of large sets of data to “train” the computational model, and in recent years we have seen an explosion in the amount of protein sequence and protein structure data. Second, DeepMind’s success relied on a combination of creative ideas, brilliant engineering, and powerful computers. The DeepMind algorithm, termed AlphaFold2, consists of several modules – interlinked computational building blocks that each perform a task. The algorithm starts with a module that searches databases with the protein sequence of interest, generating an alignment that consists of similar but not identical sequences from different organisms. Using these alignments, amino acids that change throughout evolution can be identified, and then correlations can be found between changing amino acids, which others have shown are typically in contact with one another in the folded structure. Another module searches the database of protein structures for those that are similar to the sequence of interest and generates a representation of which amino acids might be in contact with one another, called a contact map. What happens next is part of the secret sauce of DeepMind’s approach. The outputs from the previous two modules are fed into another part of the algorithm that determines which pieces of information are the most valuable, seeking to improve both the sequence alignment and the contact map. After several cycles of iteration, the refined sequence alignment and contact map are fed into a final module that constructs a three-dimensional representation of the protein. A critical feature of the DeepMind approach is that it is iterative – the final structure is fed back into the previous modules to refine the sequence alignment and contact map, which are used to produce a refined structure.
So why is the ability to predict protein structure so important? First, knowledge of the shape of a protein – its structure – many times reveals things about its function inside of cells. Second, modern drug discovery relies heavily on knowledge of protein structure, and being able to predict structure accurately from sequence promises to speed up this process. We can see how drugs fit into binding pockets and design new drugs once we understand what parts of the protein are interacting. Third, being able to predict protein structure helps us understand how disease-associated mutations might affect protein structure and function, paving the way to better understand disease and how to treat it. And finally, there is a lot of interest in designing proteins that have new functions – for example, proteins that can bind to the receptor that the SARS-CoV2 virus uses to get into cells, thereby preventing the virus from gaining access. The ability to predict structure from sequence will likely speed up the process of protein design.
For this landmark discovery, John Jumper and Demis Hassabis are being awarded the 2023 Albert Lasker Basic Medical Research Award.
References:
(1) Haber, E. and Anfinsen, C. B., J. Biol. Chem. 237, 1839 (1962).
(2) Kryshtafovych, A.; Schwede, T.; Topf, M.; Fidelis, K.; Moult, J. Proteins 89, 1607-1617 (2021).
(3) Jumper, J.; Evans, R.; Pritzel, A.; Green, T.; Figurnov, M.; Ronneberger, O.; Tunyasuvunakool, K.; Bates, R.; Zidek, A.; Potapenko, A.; Bridgland, A.; Meyer, C.; Kohl, S. A. A.; Ballard, A. J.; Cowie, A.; Romera-Paredes, B.; Nikolov, S.; Jain, R.; Adler, J.; Back, T.; Petersen, S.; Reiman, D.; Clancy, E.; Zielinski, M.; Steinegger, M.; Pacholska, M.; Berghammer, T.; Bodenstein, S.; Silver, D.; Vinyals, O.; Senior, A. W.; Kavukcuoglu, K.;Kohli, P.; Hassabis, D. Nature 596, 583−589 (2021).
(4) AlphaFold 2 is here: what’s behind the structure prediction miracle<
Acceptance remarks
Acceptance Remarks: Demis Hassabis
I am deeply honoured to receive the 2023 Lasker Basic Medical Research Award together with John Jumper. In common with almost any research breakthrough in modern science, the work is the result of a large multidisciplinary team effort and we would like to acknowledge the immense contributions of our wonderful colleagues on the AlphaFold team and also more widely across the whole of DeepMind and Google.
The journey we’ve been on has been amazing. I remember first coming across the protein folding problem 30-years ago as an undergraduate, and becoming fascinated by it, thinking how it might be the perfect type of problem to tackle with AI one day.
When we founded DeepMind in 2010, our goal was to use artificial intelligence to advance knowledge and accelerate scientific discovery. The watershed moment came in 2016 when our prior system, AlphaGo, became the first AI program to beat a world champion at the complex and ancient game of Go. I knew then we had the general AI knowhow and ideas to tackle as formidable a challenge as protein folding and the AlphaFold project was born.
It took many further years of development and innovation specific to the problem of course. AlphaFold proved to be the most difficult and complex AI system we had ever built, but when we received the results for the CASP13 competition in 2020 we knew we had achieved atomic accuracy on the target protein structures, an amazing moment that none of us on the team will ever forget.
Since AlphaFold’s release, we have been thrilled by what the scientific community has done with it. To date it has been used by over a million researchers to advance a huge and diverse range of work, everything from enzyme design to disease understanding to drug discovery. The speed with which AlphaFold has been adopted by the biological community as a standard research tool has been very gratifying to see – it is everything we hoped for and more, and hopefully just the beginning of the impact it will make.
AlphaFold is one of the first examples of what I like to call ‘science at digital speed’, both in terms of the speed of the solution (a few seconds for an average protein) and the speed of the dissemination of that solution (as fast as a keyword search on a database). We very much hope that when we look back on AlphaFold in a decade’s time it won’t just be an isolated instance of AI being productively applied to modelling complex biological phenomena but in fact the heralding of a new golden era of digital biology.